About Me
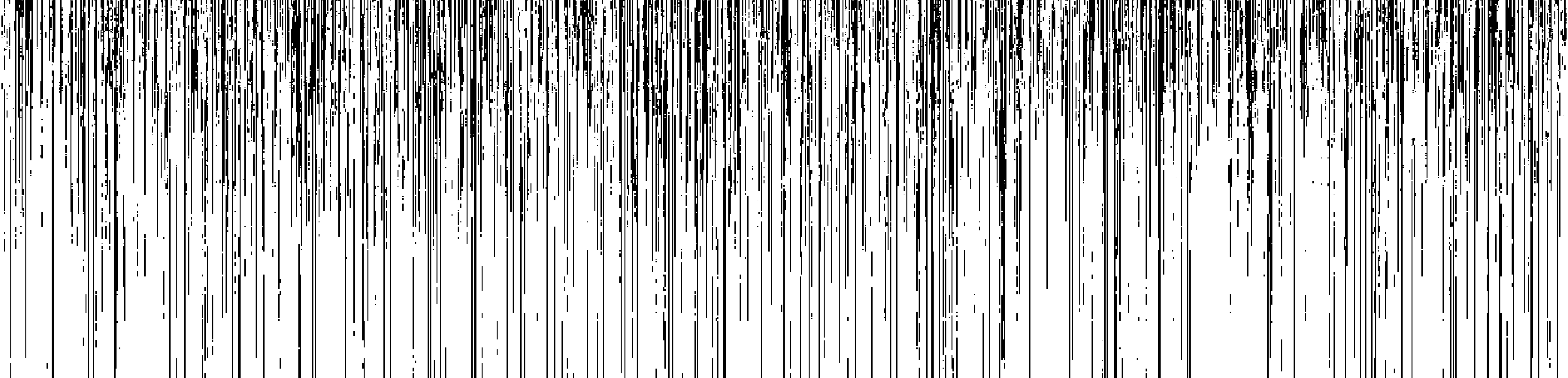
I am the Iris & Howard Critchell Assistant Professor in the Mathematics Department at Harvey Mudd College. My research focuses are in mathematical data science, optimization, and applied convex geometry. I leverage mathematical tools, such as those from probability, combinatorics, and convex geometry, on problems in data science and optimization. Areas in which I have been active recently include randomized numerical linear algebra, combinatorial methods for convex optimization, tensor decomposition for topic modeling, network consensus and ranking problems, and community detection on graphs and hypergraphs. My research is supported by NSF DMS #2211318 “Tensor Models, Methods, and Medicine”.
Before starting at HMC, I received my PhD in the Graduate Group in Applied Mathematics at the University of California, Davis where I was fortunate to be advised by Professor Jesús A. De Loera, and then was a CAM Assistant Professor (post-doc) in the University of California, Los Angeles (UCLA) Mathematics Department where my exceptional postdoctoral mentor was Professor Deanna Needell.
Recent News
July ‘24: I was named the Iris & Howard Critchell Assistant Professor. This chair is awarded to a junior professor in advance of earning tenure as a way to recognize faculty, who in the early stages of their careers, have exhibited an unusual talent for mentoring and counseling students in all aspects of their lives: curricular, extracurricular, and personal. I am honored to hold this chair named after Iris and Howard Critchell, who were founding directors of the Harvey Mudd College Bates Aeronautics Program and amazing people!
June ‘24: We (with collaborators Minxin Zhang and Deanna Needell) submitted our paper Block Matrix and Tensor Randomized Kaczmarz Methods for Linear Feasibility Problems! In this paper, we propose new block variants of the randomized Kaczmarz method for solving linear feasibility problems defined by matrices and tensors under the tensor t-product. We prove that these methods converge linearly in expectation to the feasible region. We also illustrate the effectiveness of our methods through numerical experiments in image deblurring!
April ‘24: Anna Ma and I will be mentoring a Broader Engagement Guided Affinity Group (GAG) on “Randomized Algorithms for Challenging, Big Data” at the SIAM Conference on the Mathematics of Data Science (MDS) 2024. Students in our GAG will meet with us virtually before the conference, will attend conference sessions relevant to our topic, will meet with us each morning at the conference, and hopefully will learn a lot! Anna and I are excited to meet these participants and to experience MDS 2024 with them!
April ‘24: We (with collaborators Alejandra Castillo, Iryna Hartsock, Paulina Hoyos, Lara Kassab, Alona Kryshchenko, Kamila R. Larripa, Deanna Needell, Shambhavi Suryanarayanan, and Karamatou Yacoubou-Djima) submitted our paper Randomized Iterative Methods for Tensor Regression Under the t-product! In this paper, we extend variants of the Kaczmarz and Gauss-Seidel methods to tensor regression under the t-product, which also yields novel insights into the standard matrix-vector and matrix-matrix regression settings. In addition, we survey the related work in the matrix-vector and tensor regression literature and provide a suite of numerical experiments that illustrate the strengths and weaknesses of our proposed methods, including demonstrating their application to image deblurring!
March ‘24: We (with students Nestor Coria and Jaime Pacheco) submitted our paper On Quantile Randomized Kaczmarz for Linear Systems with Time-Varying Noise and Corruption! In this paper, we consider solving systems of linear equations which have been perturbed by adversarial corruptions with the quantile randomized Kaczmarz (QRK) method. Previously, QRK was known to converge on large-scale systems of linear equations suffering from static corruptions. We proved that QRK converges even for systems corrupted by time-varying perturbations. This is an important regime as many applications where linear systems arise deal with distributed data access, and noise or corruption introduced into the system can vary across time and across data access!
March ‘24: I am serving as a SIAM representative on the Joint Taskforce on Data Science Modeling Curriculum, which is a shared effort between ACM, ASA, MAA and SIAM. The taskforce extends the efforts of the ACM Data Science Task Force towards a complete data science model curriculum, towards a multidisciplinary effort with representatives from computing, statistics, applied mathematics, and other possible societies. Excited to be a part of this great effort!
February ‘24: Our (with collaborators Bryan Curtis, Luyining Gan, Rachel Lawrence, and Sam Spiro) paper “Zero Forcing with Random Sets” appeared in Discrete Mathematics! In this paper, we investigate the probability that a randomly sampled set of vertices of a given graph (each vertex included in the set independently with probability p) will serve as a zero forcing set for the graph. This work additionally resolves a conjecture of Boyer et. al.