About Me
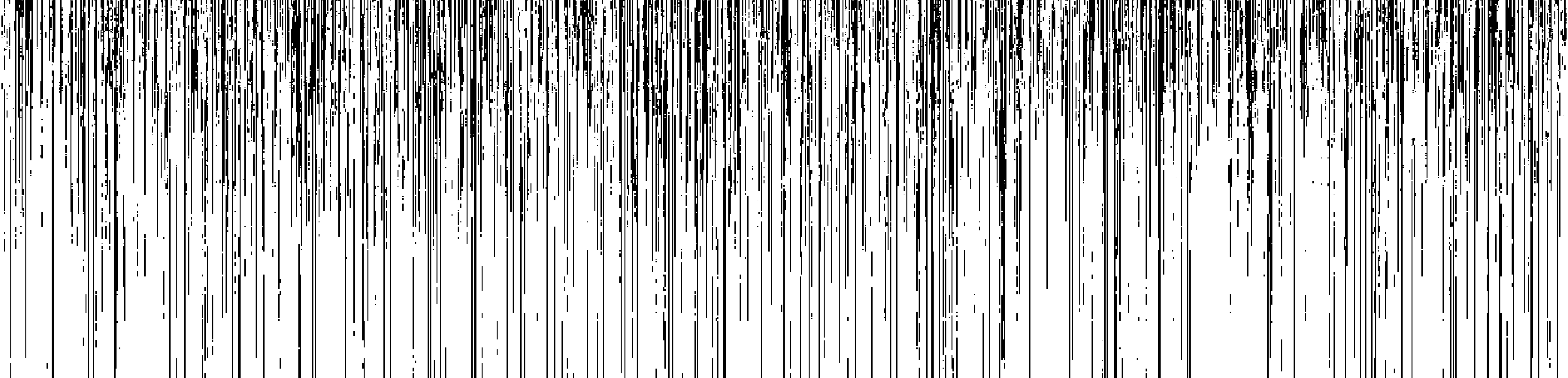
I am the Iris & Howard Critchell Assistant Professor in the Mathematics Department at Harvey Mudd College. My research focuses are in mathematical data science, optimization, and applied convex geometry. I leverage mathematical tools, such as those from probability, combinatorics, and convex geometry, on problems in data science and optimization. Areas in which I have been active recently include randomized numerical linear algebra, combinatorial methods for convex optimization, tensor decomposition for topic modeling, network consensus and ranking problems, and community detection on graphs and hypergraphs. My research is supported NSF CAREER #2440040 “Randomized Iterative Methods for Corrupted Data, Constrained Problems, and Compressed Updates” and has been supported by NSF DMS #2211318 “Tensor Models, Methods, and Medicine”.
Before starting at HMC, I received my PhD in the Graduate Group in Applied Mathematics at the University of California, Davis where I was fortunate to be advised by Professor Jesús A. De Loera, and then was a CAM Assistant Professor (post-doc) in the University of California, Los Angeles (UCLA) Mathematics Department where my exceptional postdoctoral mentor was Professor Deanna Needell.
Recent News
March ‘25: We (with collaborators Alejandra Castillo, Iryna Hartsock, Paulina Hoyos, Lara Kassab, Alona Kryshchenko, Kamila R. Larripa, Deanna Needell, Shambhavi Suryanarayanan, and Karamatou Yacoubou-Djima) submitted our paper Block Gauss-Seidel methods for t-product tensor regression! In this paper, we extend two variants of the block-randomized Gauss-Seidel method to solve a t-product tensor regression problem. We additionally develop methods for the special case where the measurement tensor is given in factorized form. We provide theoretical guarantees of the exponential convergence rate of our algorithms, accompanied by illustrative numerical simulations.
March ‘25: We (with collaborators Alejandra Castillo, Iryna Hartsock, Paulina Hoyos, Lara Kassab, Alona Kryshchenko, Kamila R. Larripa, Deanna Needell, Shambhavi Suryanarayanan, and Karamatou Yacoubou-Djima) submitted our paper Quantile-Based Randomized Kaczmarz for Corrupted Tensor Linear Systems! In this paper, we develop a Quantile Tensor Randomized Kaczmarz (QTRK) method robust to large, sparse corruptions in the observations of a tensor regression problem under the t-product. This approach combines the tensor Kaczmarz framework with quantile-based statistics, allowing it to mitigate adversarial corruptions and improve convergence reliability. We also propose a masked variant, which selectively applies partial updates to handle corruptions further. We present convergence guarantees, discuss the advantages and disadvantages of our approaches, and demonstrate the effectiveness of our methods through experiments, including an application for video deblurring.
March ‘25: Our paper (with students Zane Collins, Tyler Headley, and Luke Wang) paper Quantile Multiplicative Updates for Corruption-Robust Nonnegative Matrix Factorization was accepted to the Sampling Theory and Applications (SampTA) conference in Vienna, Austria in August 2025! In this paper, we introduce a quantile-based variant of the popular multiplicative updates method for training the Frobenius norm-formulation of NMF which avoids the effects of corruption in the data. Our numerical experiments illustrate the promise of this method, and show that in some scenarios, this method applied to the corrupted data recovers factorizations nearly as good as those learned on the uncorrupted data!
February ‘24: I was recently awarded an NSF CAREER award for my project “CAREER: Randomized Iterative Methods for Corrupted Data, Constrained Problems, and Compressed Updates”! This project will support undergraduate researchers and visiting graduate researchers at Harvey Mudd College each of the next five years. Our work will focus on randomized methods in the presence of adversarial perturbations to input data, problems with challenging solution constraints, and in the extremely large data regime. Additionally, the project will support the development of course materials for a new course in numerical linear algebra and an annual workshop for faculty at primarily undergraduate institutions (PUIs) interested in effective undergraduate research mentorship. This was covered by a very nice story from Harvey Mudd College communications!
January ‘25: My group’s (with Anna Ma (UCI)) proposal was accepted to the Institute for Advanced Study (IAS) Summer Collaborators program! We will be visiting for a two week-long research visit in July 2025, where we will be developing methods for large-scale linear systems that require only partial information. We are very excited for this opportunity!
December ‘24: We (with collaborators Alejandra Castillo, Iryna Hartsock, Paulina Hoyos, Lara Kassab, Alona Kryshchenko, Kamila R. Larripa, Deanna Needell, Shambhavi Suryanarayanan, and Karamatou Yacoubou-Djima) submitted our paper Randomized Kaczmarz methods for t-product tensor linear systems with factorized operators! In this paper, we extend the randomized Kaczmarz method to solve a t-product tensor system where the measurement tensor is given in factorized form. We develop variants of the randomized factorized Kaczmarz method for matrices that approximately solve tensor systems in both the consistent and inconsistent regimes. We provide theoretical guarantees of the exponential convergence rate of our algorithms, accompanied by illustrative numerical simulations, including in image deblurring!
October ‘24: I am a SIAM representative on the Joint Taskforce on Data Science Modeling Curriculum, which is a shared effort between ACM, ASA, MAA and SIAM. The taskforce extends the efforts of the ACM Data Science Task Force towards a complete data science model curriculum, a multidisciplinary effort with representatives from computing, statistics, applied mathematics, and other possible societies. Our work on the forthcoming ACM-ASA-MAA-SIAM++ Competencies for Undergraduate Data Science Curricula was featured in SIAM News!